A data strategy helps companies know how to manage and analyze data to confront challenges and seize opportunities to thrive. In this blog, you will learn data strategy definition, importance, goals, components, and tips on developing a data strategy that takes your business’s revenue to the next level.
What Is A Data Strategy?
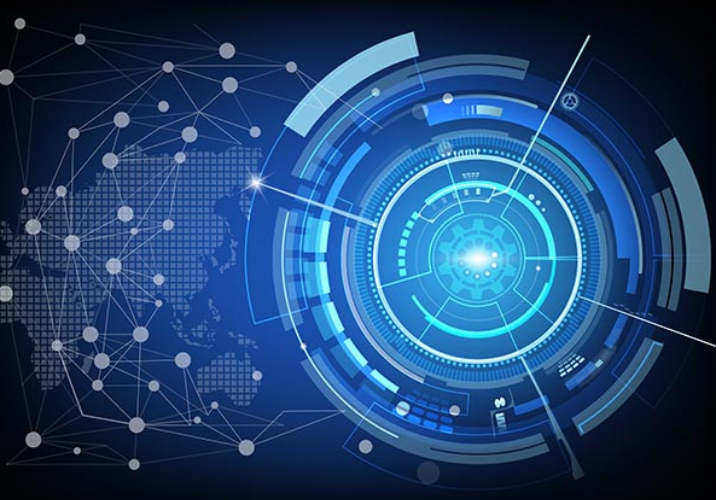
A data strategy is a roadmap or a framework that defines how the data is collected, stored, utilized, managed, and shared in a company. Generally, a data strategy’s main purposes are:
- Explain how the company meets its goals by completing the desired data activities.
- Describe what changes are necessary for the company to optimize the value of its data activities and outline plans for it.
- Create a timeline for completing the planned activities, define milestones, and a strategy to move forward.
Why Does Data Strategy Matter?
Traditionally, data was considered a byproduct of business activity or process. It provided little value after the process was finished. While there might have been one or more other applications that needed to approach the content for follow-up (special reports, customer service, audits…), these were often one-off activities.
Business is now different. The value of data is appreciated. The results of analytics and reporting have made data essential in various new business initiatives. It’s popular for application data to be shared with a maximum of 10 other systems.
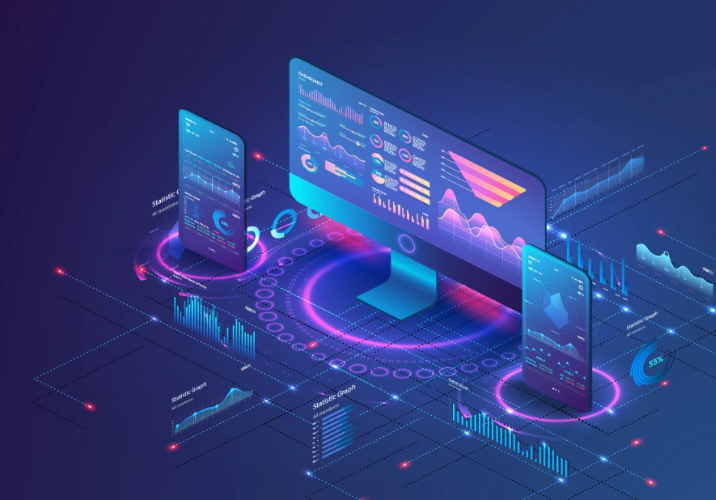
A good data strategy can help companies solve the following problems:
- Business procedure is not efficient and does not utilize all resources.
- Data analysis is hindered by certain data issues: data quality, data privacy and data integrity.
- There is insufficient understanding of why some departments are getting left behind in terms of efficiency. (supply chain, customer retention…)
- Multiple departments have duplicated data.
- Data access is not easily granted for different departments.
- The current business needs and goal are not clear.
What Are Data Strategy Goals?
To address the above problems, you need to set goals first. Since the IT department is in-charge of implementing the framework and tool for your data strategy, you should consider your IT resources to set feasible goals:
- Innovation: Developing a data strategy means that your company is heading towards innovation.
- Meet the requirements of employees: A data strategy should aim to serve and support employees within the company.
- Address risk and regulations: A good data strategy should be able to solve data security risks and data compliance requirements.
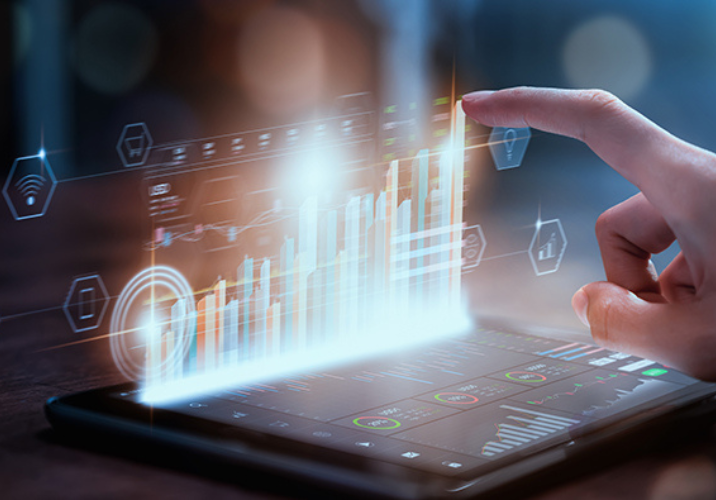
Three Components of A Data Strategy
Business Strategy
The ultimate goal of data strategy development is to serve your business strategy. You should align your business goals and processes when building your data strategy. Even when there is already a data strategy for your company that existed years ago, you should review and make timely changes to ensure it is well cooperated with the current business strategy. (Because the tools and timeline for data analysis and management could be outdated)
Organization Roles
Not all people use and work with data the same way, that’s why you need to set roles to clearly determine the positions and responsibilities of members. This is crucial for data security and management.
- Data engineers: supervise the data pipeline and build data architecture.
- Data scientists: work with data that the pipeline delivers
- Data analysts: analyze and interpret data
- Business managers: manage data operations and review reports.
Data Architecture
Data architecture includes processes and tools (both on-premises and cloud-based) that help you transform and analyze data. There are three stages in building a data architecture:
- Data identification: To define your data architecture, you need to know what the current datasets are. You can review data sources with the IT team and data team or the users who work with data frequently or you can also use data catalog tool.
- Data ingestion and storage: Structured and unstructured data from multiple sources and applications need to be centralized and processed in data warehouse or data lake. This is the initial step to format data and prepare for data analysis.
- Data analysis: Data can be visualized into comprehensive graphs to uncover trends and patterns. Predictive and prescriptive analytics can also be used to identify problems and forecast goals.
Among the three data strategy components, data architecture is a major foundation to good and consistent data strategy, which is helpful when your data operation scales up in the future.
What To Consider When Creating A Data Strategy?
There is a general belief that only large enterprises with huge volumes of data benefit from a data strategy. However, a good data strategy can greatly support small businesses, helping them set the foundation for scaling.
Although the value of data has expanded immensely for 20 years – and business users realize it, several companies have changed their approaches to collecting, sharing, and manipulating corporate data assets. Their behavior shows an outdated, hidden belief that data is just an application byproduct.
Businesses need to map out data strategies that match today’s realities. To construct such a thorough data strategy, they need to ponder over current business and technology commitments while also attaining new objectives and goals.
Here are three criteria you should consider before crafting your data strategy:
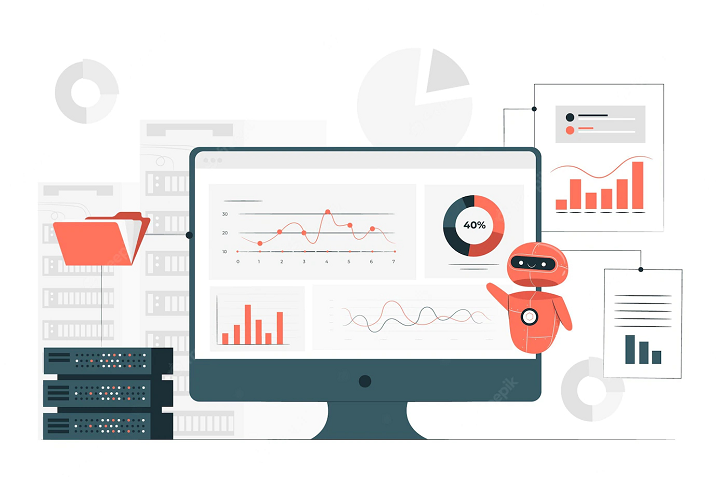
Your Data Maturity Level
First and foremost, a self-assessment is essential for establishing your data strategy.
Where does your business fall in the data maturity stage?
For example, Dell has a broadly used “Data Maturity Model” that allows companies to explore how data-driven their business is. The model involves four stages, including:
- Data aware – Your business has not standardized its reporting system and there’s no integration between your systems, databases, and data sources.
- Data proficient – There is still a lack of trust in the data, especially its quality. You may have a data warehouse, but there are still some pieces lost.
- Data savvy – Your data empowers your enterprise to make business decisions. However, there are still some issues between business leaders and IT because IT works to offer trusted data on demand.
- Data driven – IT and business are cooperating and staying on the same page. Now, the concentration is on developing the data strategy as the foundation work (especially integrating data sources) has been successfully performed.
What’s most vital here is being realistic about where your business falls. It seems to be insufficient to look at your feelings about how data-driven your brand is. You need to look at the facts. Begin by discovering the data issues your business currently encounters, as that is a good indicator of where you stand.
Your Company and Industry Size
There are two approaches when it comes to data strategy framework:
- Data defense: focuses on things such as data access, security, governance, and accuracy
- Data offense: refers to collecting insights for decision making.
Every business needs a balance of defense and offense. However, depending on their industry, some will focus more on one end of the spectrum.
Let’s see two data strategy examples:
Example 1: a financial institution or healthcare organization often processes highly sensitive data, where data security and privacy are prioritized. It’s not a top priority to collect real-time data and fast insights but setting access permissions is paramount. In this case, they will concentrate more on a defense framework.
Example 2: the technology industry tends to change swiftly and relies heavily on a rapid turnaround of data insights. Hence, they lean more on offense. That being said, there are specific departments in tech companies that will lean more on defense, like finance.
Your industry and your company’s size will decide whether you choose a centralized or distributed approach for your data strategy. The framework you select will show which strategy works best for your business.
- Centralized model: You’ll get a centralized reporting or business intelligence team that handles and processes the data and the reports.
- Distributed model: It is suitable for larger teams who choose the offensive approach. Every team can move rapidly and is supported to accomplish their work effectively. In this model, business intelligence takes charge of the platforms and sets the guardrails while teams handle the development work.
Your Data Management Team
Data science is a hot topic in data management. Glassdoor has listed it as the second-best job in the US in 2022. However, if you’re wondering what position to add to your management data, a data scientist might not be your first option.
Suppose you are in the earlier stages of the data maturity model. In that case, you might need a data warehouse architect or a data analyst who is familiar with writing SQL and creating SQL tables.
Regarding the more technical tasks such as putting data in the warehouse, there are many third-party tools to help you. At this stage, all you need is a person that can aid you in structuring your data.
Suggested for you: Data Monetization: A Complete Guide | |
How To Develop A Data Strategy in 7 Steps
To form a feasible data strategy, you need to follow 7 steps. Also, you’ll find instructions on how to do each step below.
Step 1: Make a Proposal and Seek Approval
The first and foremost stage in establishing a data strategy is to create a proposal for creating a data strategy and submit it for approval. Having your proposal approved by personnel at all levels is essential because you’ll need their participation for the strategy to be successful.
To win approval from leadership, you’ll have to prove the strategy will do wonders for the business and how. It is vital that you include economic logic in your report. Also, illustrating how your rivals are utilizing data to better their business is a plus. You should also use compelling examples and data to support your claims.
You’ll have to receive approval from leaders of departments, technology partners, and other employees who will utilize or contribute to managing data onboard as well. Remember that giving people a sense of ownership over the process will help improve your results significantly.
Getting approval can be time-consuming to some extent. You may need to review and adjust your proposal several times to fix the possible flaws and make it more convincing.
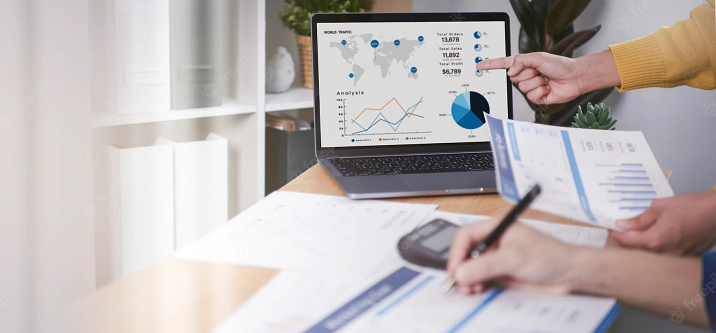
Step 2: Build a Team to Manage Data and Appoint Data Governance Tasks
Once you’ve obtained adequate approval, you can jump to creating a data management team. The team should consist of senior managers and department heads from various parts and units, who are aware of the value of data and challenges relating to data.
They also have a deep understanding of the company’s technological and organizational competence, as well as its opportunities and limitations. This team’s responsibility is allocating resources, implementing and upgrading policies, as well as handling data-related tasks and possible issues.
When the team is fully formed, you’ll appoint data governance roles to the members. The crucial roles are mainly ensuring everything is standardized, deploying technologies, informing personnel about updates in policy, and more. Having these roles well assigned will boost the productivity of the team and create a deep sense of ownership among them.
Step 3: Define the Types of Data You Want to Collect and Their Sources
What you need to do next is to identify the types of data you need and how to gather it. You’ll do this in accordance with your business goals.
For example, if you’re a blogger and you want to optimize your posts to be in the topics your audience is fondest of, you’ll need to gather data of their interest and the types of posts they read. You can do this by observing which posts different groups of the audience click on or browsing your viewers’ social media profiles to see which pages they like, which community groups they’re in, and what topics they usually post about.
Step 4: Identify Objectives for Data Collecting and Distributing
The keystone of an effective data strategy is having specific objectives. You ought to create both long-term and short-term objectives, as well as both overarching objectives and ones that apply to single tasks and departments.
Specifically, describe how data can contribute to attaining goals of the business as a whole and how each department can benefit from it. That’s called long-term objectives of a data strategy. And for short-term objectives, define ones for establishing policies regarding the gathering, storage, usage, and sharing of data.
Each department can set objectives for how they are going to utilize data as well. Thanks to this, they’ll be able to keep tabs on their progress and report to the data management team. This is with the aim of providing insights into how data is used across the company.
Step 5: Develop a Data Strategy Roadmap
When the objectives are already outlined, you’ll need to launch a plan for how to materialize them. This plan is called a data strategy roadmap.
Your data strategy roadmap should consist of who owns the objective, what process and technology they will adopt, the expenses, the estimated time to fulfil and the expected results. During the process of the projects, you should regularly revise them to make evaluation to see if it’s working well.
Step 6: Schedule for Data Storage and Organization
Data storage and organizing are chief components in a data strategy since they aid in defining how sharable and actionable your data is.
Data storage is simple, whereas data organization might be distinct across companies. When developing plans for data storage, how much storage capacity should be considered. Besides, you should also consider how your storage method will affect data sharing and usage. How you organize data decides how accessible, understandable, and usable it is. Your storage approach impacts how easy it is for different parts of the company to share data.
Step 7: Attain Approval From the Leadership and Start Adopting Your Data Strategy
Once you have all your objectives mapped out, it’s time to submit in the form of a business plan to the company leadership for approval.
To make sure you get approval, you should include the strategies you’ll employ to reach the company’s data goals in the business plan. Plus, there should be the resources you’ll need to carry out the strategy, as well as capital investments, new personnel, new processes, and new organizational frameworks.
After earning the approval from the leadership, you can start the data strategy. As said before, you should revise the strategy frequently to make timely adjustments and improvements if needed.
4 Pitfalls to Avoid When Developing A Data Strategy
Pitfall 1: Data is the Last Thing You Think About
In many organizations, a data team is always put into a reactive situation. If any problem arises, a new strategy developed, they are the last to hear about it. Then, they’re urged to brainstorm, pulling reports, plans, tactics — anything that might be useful — out of nowhere. Data analytics teams sometimes find themselves as a help centre, rather than contributing to defining strategy from the outset.
Thinking about data last is a dangerous trap, especially for companies that are developing fast. In fact, it ensures that the data teams get caught on the back foot with nowhere to turn — until someone breaks the cycle.
Solution:
- Raise the question early and often when it comes to data. And when talking to leaders across the company, make sure you connect the dots between the data and business outcomes:
- Target: CRO. Strategy: list out the impact of healthy CRM data on shortening sales cycles and boosting cross-sell and upsell.
- Target: COO. Strategy: express the influence that ineffective data processes are having on within the organization, and recommend practical solutions for time and budget saving.
- Target: CMO. Strategy: express that you understand the vital function of customer data in marketing campaigns, and emphasize that gathering quality customer data requires well-built data architecture.
Pitfall 2: Overestimate Your Data Capability
Everyone wants to create a data-driven culture, of course – but how realistic is that goal? It is true that many of us wish to utilize data to make any decision, or data-driven decisions, not every organization can allocate enough time, budget, and have the motivation to materialize it.
According to research by McKinsey Analytics, 61% of organizations that acknowledge the impact of data and analytics on core business practices are still adopting an ad-hoc method for analytics rather than building a full-fledged data strategy.
Solution:
Ensure that every meeting related to markets should start with reviewing metrics; and every program should be assigned leading and lagging indicators for measuring effectiveness.
To go further, you might want to show live feeds of particular key reports in your organization messaging center. You can also send regular monthly or quarterly updates from each line of business to all employees, so everyone has a chance to update themselves of the latest advancements. If there are a layer of meta-analysis for the data, they’ll enjoy the certainty that the reported numbers are accurate and up-to-date.
Pitfall 3: Unable to Think From the First Principles
Regarding data, it is extremely easy to lose track of what really matters. Many companies usually overfocus on irrelevant metrics, working around sophisticated dashboards whose chart dramatic changes over time without ever solving single problem about the core business. Have you ever found yourself staring at some figures and metrics and wondering what they even mean? And if you have, how often?
This mindset nurtures a tendency to invest in the latest tools without setting up a solid foundation first.
Solution:
A clear strategy is what you need to get started.
Spend time listing questions that you want to find answers to. If your main goal for sales is to get as many quality leads as possible, website traffic alone isn’t going to reveal much about how people enter the sales funnel or where and why they leave. Instead, write a list of things you wish you could know.
Just like that, make sure you understand what problems you want to solve before start using a new tool.
Allocate time and effort to arouse the enthusiasm around the initiative – not just in your team, but within other teams who may use the tool for some purposes. Provide training programs for new users and refresh regularly to keep adoption and enthusiasm high.
Pitfall 4: Let Data Silos Take Control
No organization can totally avoid the occurrence of data silos. Even the youngest, smallest ones may have two engineers running applications on different servers. As long as everything is functioning and everyone knows where to find what they need, silos aren’t necessarily a problem. However, when confusion, black holes, and bottlenecks can occur due to silos, it’s time to take measures.
An easy strategy often seems like a good way to handle not so complicated problems, but that doesn’t always mean it’s the most viable solution. For example, let’s say your marketing department wants to launch a new on-page SEO tool — but it has no integration capabilities with your other data systems. If there are only five users of that tool today, shouldn’t that be alright? Not really. Buying a new tool may be easy, but you may create plenty of work down the road when that data becomes vital for other departments of the company.
Solution:
If there has to be any inefficiency in your company, it’s highly likely to be data silos. Instead of looking for silos, find out what the real problems are. When you’ve already known what problems you’re trying to solve, break it down into manageable steps — and if that includes changing technology to eliminate a silo, make sure that everyone understands those long-term advantages and try to get them eager about the change.
As new needs appear and gaps become significant, Synodus’s data experts can offer a method for discovering the necessary changes across your organization’s different data management abilities and technology areas. With the help from our team of experienced data analysts and data scientists, you can develop a data strategy that acts as a roadmap to meet both current and upcoming data management needs. | |
Conclusion
The idea behind a data strategy is not to create a perfect world that can handle any unexpected data demand. Instead, it helps position you to bring the best possible solution when your company’s requirements increase and evolve. We hope that you can clearly understand data strategy meaning, importance, components, and how to build a data strategy for your company.
More related posts from Big data blog you shouldn’t skip: