Sales forecasting has a substantial role in every business’s success when making informed decisions. Yet, most predictions are usually less than 75% precise. In this case, Predictive Analytics technology can help us make the most tangible strategy based on data and reasonable information. This article will demonstrate how to build a reliable sales forecast using Predictive Analytics in Excel. It is a cost-effective option that leverages historical sales data to anticipate future sales.
What Is Predictive Analytics
Predictive analytics forecasts future events based on present and historical data. It used various techniques, from data mining to extract information to artificial intelligence (AI), machine learning, or modeling to spot patterns and generate suggested outcomes.
How Can Predictive Analytics Be Useful For Sale Forecasting
Predictive analytics is an excellent solution for risk management, planning, and budgeting tool. As companies estimate sales using data, they are mitigating some of the human biases that can negatively affect business development and sustainability. Business owners might be too optimistic about the future and make bad decisions based on emotions rather than educated predictions.
Moreover, if companies are excessively conservative and cautious, they might miss precious chances to boost growth rates or develop business operations. Predictive analytics can stimulate business owners to get ahead in a new trend to quickly stock and sell products or grow current sales teams to meet the demands.
Furthermore, predictive analytics also spot minor abnormalities and urge businesses to solve them before they become big problems. For instance, your forecast might show early warning signs about your sales pipeline that can be solved and adjusted.
With the information from a reliable sales forecast, you can make the right business operations decisions. Thus, predictive analytics for sales forecasting is essential for a company’s growth rate and extension potential.
Ways To Deploy Excel Predictive Analytics For Sale Forecasting
Method 1: Use Forecast Sheet
1. Five set-up steps
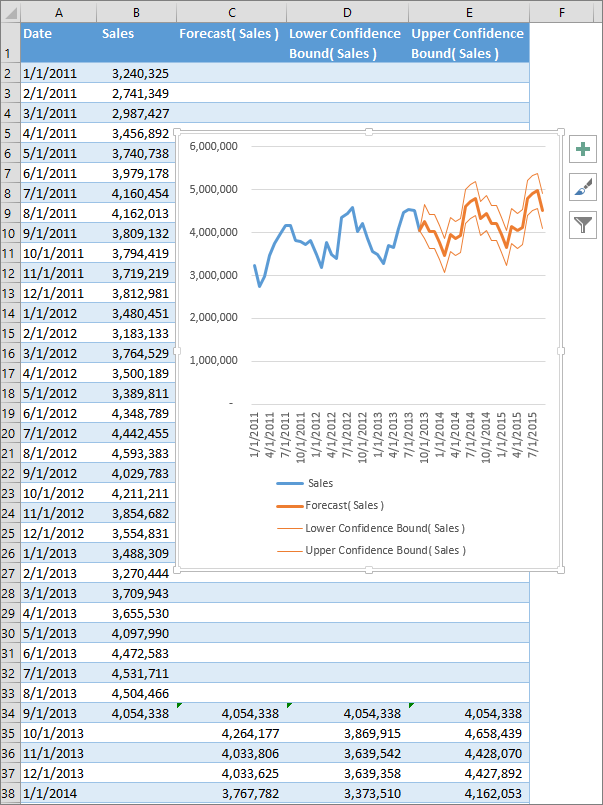
Business planning doesn’t need to be as precise as accounting and depends more on educated estimation. A great combination of educated guesses and data will give you the basis for creating a sales forecast. Now we’ll find out how to use Excel to forecast your business sales.
Step 1: Enter data
Within a worksheet, insert two data series corresponding to each other:
- A series with time entries for the timeline
- A series with corresponding values that will be forecasted for the future
Notice: The timeline requires constant intervals between its data points, such as monthly intervals with values on the first day of each month. It’s acceptable if your timeline series miss up to 30% of the data points or contains some numbers with the same timestamp. The forecast will still be precise. Nevertheless, encapsulating data before you generate the forecast will bring in more reliable forecast results.
Step 2: Choose both data series
If you choose a cell in one of your series, Excell automatically picks the rest of the data.
Step 3: Choose Forecast Sheet
On the Data tab, click on Forecast Sheet in the Forecast group.
Step 4: Choose your representation
With the Create Forecast Worksheet box, select a column chart or a line chart for the visual display of the sales forecast.
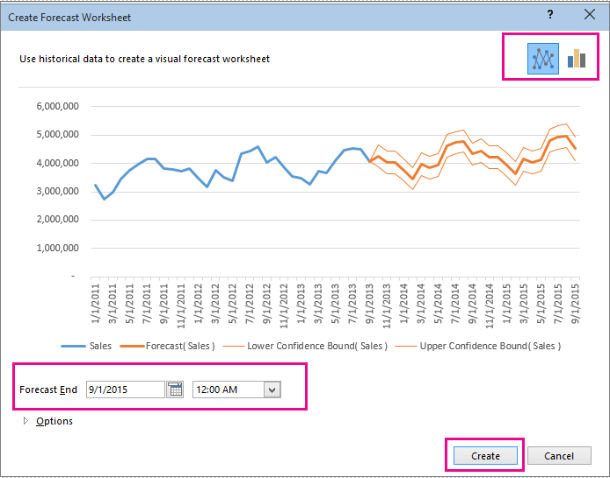
Step 5: Choose an end date
Choose an end date within the Forecast End box and click on Create.
As you build a sales forecast, Excel generates a new worksheet that includes a table of the historical and estimated values and a chart that displays this data.
You’ll see the new worksheet to the left (in front of) the sheet where you inserted the data series.
2. How to Customize Your Sales Forecast?
If you want to edit any advanced settings for your sales forecast, click on Options. Then you will find information about every forecast option.
- Forecast Start
Select the date for the sales forecast to start. As you choose a date before the end of the historical data, only data prior to the beginning date are applied in the prediction.
If you start your sales forecast before the last historical point, you’ll have a sense of the prediction precision because you can compare the estimated series to the actual data. Meanwhile, if you start the forecast too early, the prediction won’t show the forecast you’ll have using all historical data. All your historical data can bring you a correct forecast.
If your data is season-based, you should start a forecast before the last historical point.
- Confidence Interval
Check or uncheck Confidence Interval to display or hide it. The confidence interval refers to the range surrounding every predicted value, in which 95% of future points are estimated to fall, depending on the forecast with a regular distribution.
Confidence interval allows you to find the precision of the forecast. A small interval means more confidence in the prediction for the specific point. You also can use the up and down arrows to edit the default level of 95% confidence.
- Seasonality
Seasonality refers to a number for the length (number of points) of the seasonal pattern and is automatically identified. For instance, in an annual sales cycle, with every point showing a month, the seasonality will be 12. It’s possible for you to override the automatic detection by selecting Set Manually and choosing a number.
Notice: When you set seasonality manually, you need to avoid a value for less than 2 cycles of historical data. With less than 2 cycles, Excel cannot detect the seasonal components. And when the seasonality is not important enough for the algorithm to discover, your sales forecast will turn to a linear trend.
- Timeline Range
Adjust the range used for your timeline here. This range must match the Values Range.
- Values Range
Edit the range for your value series here. This range must be the same as the Timeline Range.
- Fill Missing Points Using
Excel employs interpolation to deal with missing points. That means a missing point will be completed as the weighted average its nearby points provided that fewer than 30% of the points are missing. If you want to treat the missing points as zeros, choose Zeros in the list.
- Aggregate Duplicates Using
As your data includes different values with the same timestamp, Excel will automatically average the values. To choose another calculation method, such as Count or Median, you can select the calculation from the list.
- Include Forecast Statistics
If you want extra statistical details on the sales forecast added to a new worksheet, tick this box.
Method 2: Create a Linear Regression with Analytics ToolPak in Excel
1. What is Liner Regression
This statistical approach modelizes the relationship between dependent variables (the ones you want to predict) and independent variables (the ones used for predicting). Linear regression forms an equation like this:
Y=M1*X1+M2*X2+………….+MnXn+C
We have Y as dependent variables, X as independent variables, M as the coefficients, which are the important weights assigned to the features, and C as the constant, which is the intercept. The most common technique to perform regression is the Ordinary Least Squares (OLS). It aim to reduce the sum of squares and produce the line of best fit, for instances:
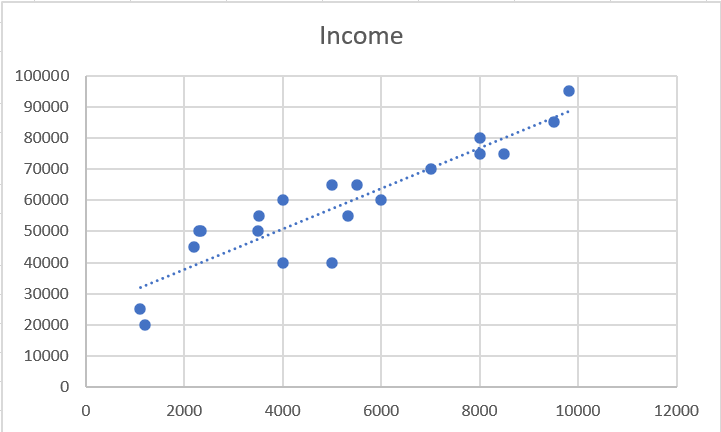
2. Open All-Important Analysis ToolPak Excel Add-in
In this second method, we use Excel Analysis ToolPack Add-in, which brings more accuracy than the above method.
- Open Excel
- Go to Files -> Options
- From the left panel, choose Add-ins
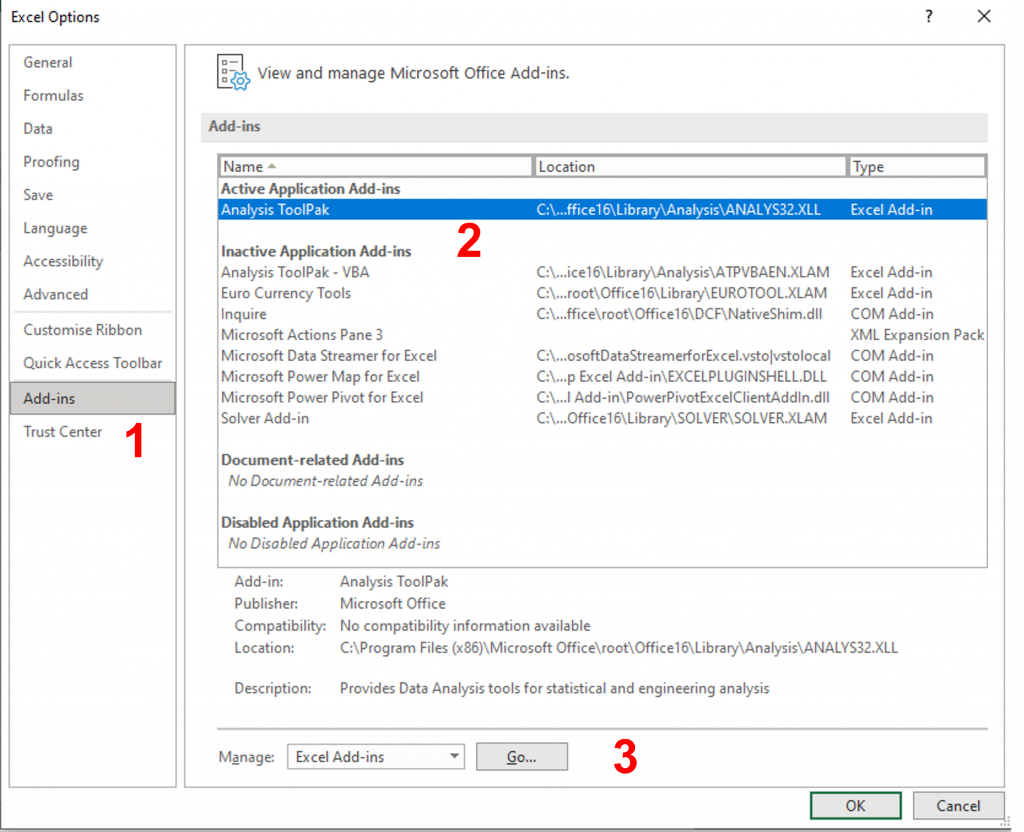
Select the “Analysis ToolPak”. This is the display of the Excel Analysis ToolPack Add-in:
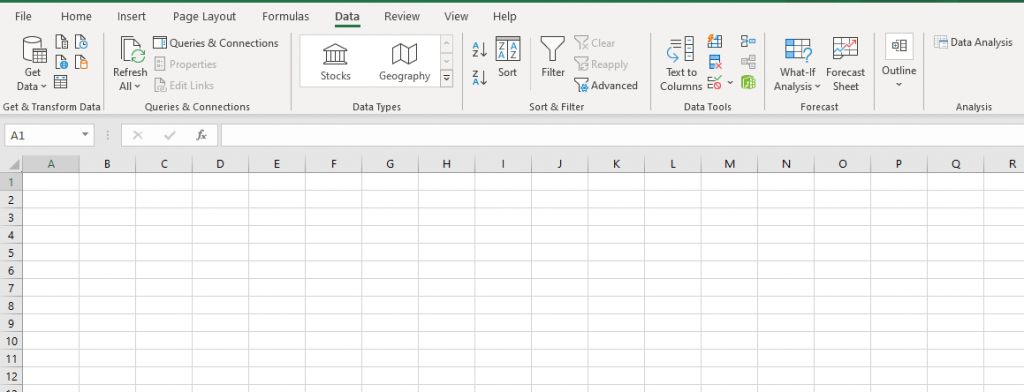
3. Implementing
To make the process easier, let’s imagine a shoe-selling company wants to predict each customer’s sales by their income, distance of home from the store, and their running frequency per week.
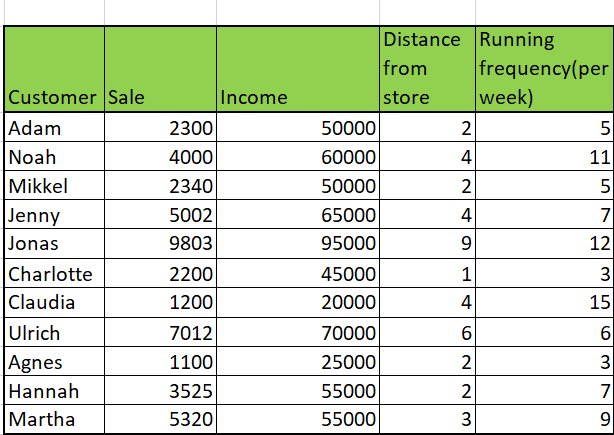
First: Encode the data to perform the regression analysis: Assigning numeric value to categorical data set
Second: Adopt Regression:
- Go to Data -> Data Analysis:
- Go to Data Analysis in the ToolPak, and select regression.
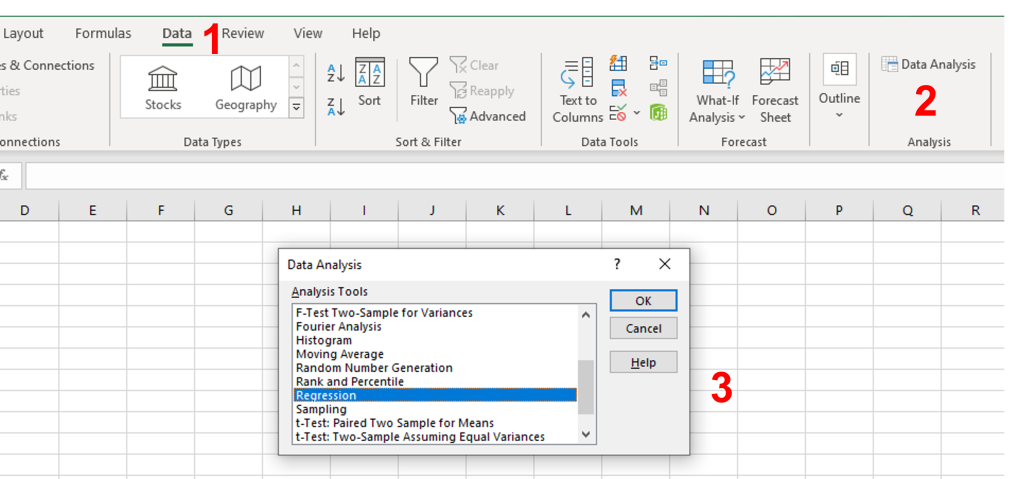
Third: Select the options:
- Input y range for the range of independent factors
- Input x range for the range of dependent factors
- Output range for the range of cells for the results
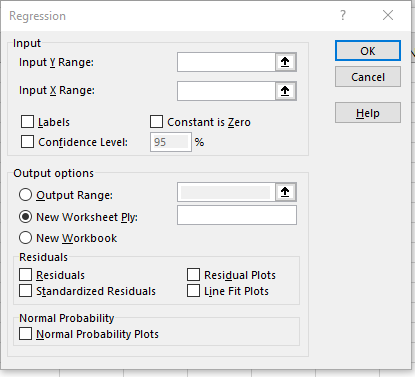
4. Complete your regression
There are 4 types of output:
- Regression statistics table
The regression statistics table defines how the line of best fit explains the linear relationship between dependent and independent variables. We have two important measures, the R squared, and Adjusted R squared values.
The R-squared statistic indicates the goodness of fit, which is how much variance can be explained by the line of best fit. The R-squared value should range from 0 to 1.
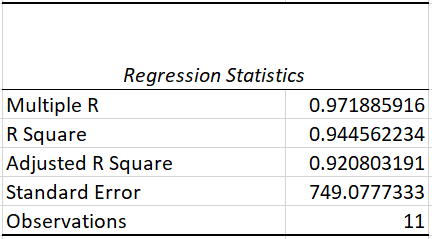
However, when adding more variables, the R squared value can increase even though the variable has no impact. Adjusted R-squared can solve this problem and show a much more reliable metric.
- Regression coefficients table
The Coefficient explains the regression line’s components in the form of coefficients.

- Residual Table
The residual table determines how much predicted value varies from the actual value.
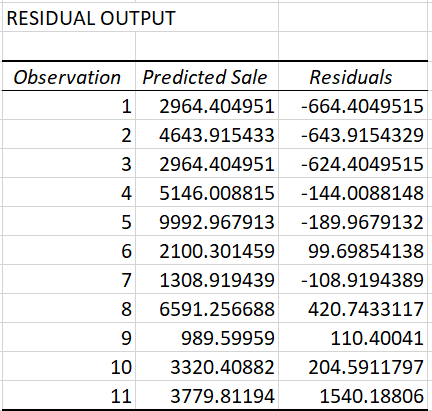
- ANOVA Table
ANOVA (Analysis of Variance) table breaks down the sum of squares into its components, which give detailed variability within the model.

Significance F (or the P-value), a fundamental metric of Anova, tells us whether your model is statistically significant or not. In other words, are your results based on randomness, or is there an underlying cause. The threshold for p-value is 0.05. If you are getting less than this, you are good to go. If not, you need to choose another set of independent variables.
5. Make prediction
Now that your regression analysis is ready, it’s time to create predictive analytics in excel. Look at this example:
You want to predict the sales of a customer. He has 40k of income monthly and lives 2km away from the store.
First, you plug in the number from the data in the linear regression model. The equation becomes:
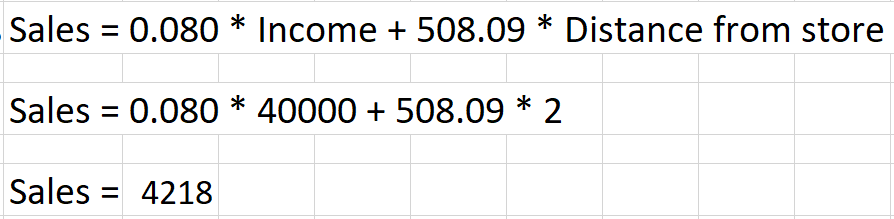
The final estimation is that the customer would pay 4218 for the products.
Source for the example: AnalyticsVidhya
The Downsides Of Using Excel For Data Analytics
Excel can be a viable choice for small businesses since it’s affordable and practical. Before deciding to use excel, keep in mind there are tools and vendors out there that help complete your predictive analytics model. Also, watch out for these limitations of excel in data analytics.
1. Excel Is Not Flexible Enough For Unstructured Data
The title said it all. Thus, to best use excel, businesses need to structure and label their database. This takes time and effort; it won’t be easy for people with no data background. On the contrary, many data analytics tools can quickly identify and analyze this data type.
One thing to note, mobile data, apps, and IoT devices usually use JSON data format, which excel finds the most challenging to work with.
2. Excel Does Not Offer Interactive Dashboards
Interactive features allow you to drill down to details with your data analytics. This works best with many data points and categories. For example, sale volume during the last 3 years, but you want it to be divided into months for comparison.
Excel provides data visualization, of course, but they are usually static and sometimes not 100% effective in demonstrating metrics. They can only show high-level trends, so it can be challenging if you look for details. In this case, you need to split your data into small charts, which can cause a countereffect of making it complicated to analyze.
3. Excel Can’t Update In Realtime
To make excel data analytics work, you must constantly update it by hand. The time you spend importing files and building worksheets, your data becomes outdated since it doesn’t connect to a live data warehouse. This is a significant disadvantage. While Excel can connect to external data sources with plugins, a data tool can seamlessly connect to dozens of sources via a data warehouse.
Nestlé Marketing team saves up 80% of time spent doing data reports after using analytics tools. Now they can do more impactful tasks for their team instead of dealing with numbers daily.
4. It Might Be Challenging To Teamwork With Excel
Imagine every department of your company has a spreadsheet with a different design, format, and template. It might not be a big deal if your company’s size is small, and every department works closely. Yet, as the company grows, it can strike a big problem of missed communication and synchronization. And while Excel offers cloud access, it’s fundamentally designed for the individual. This results in endless duplication of efforts and creates a mess.
For example, marketing teams usually need data shared from sales. With a huge database and hard-to-read templates, marketing might not get the most out of the data and effectively analyze it.
5. Security Risks Are High With Excel
Using Excel for data analytics, businesses can lose visibility into how employees use or share the data. This causes a lack of security, leading to vulnerabilities, lost data, and misuse. Unlike BI tools, Excel doesn’t require SQL coding expertise, which is why businesses continue to use it—despite the risks.
Leveraging Predictive Analytics from scratch might be a daunting start, so it would be better to seek advices from data scientists and data analysis before execution. Synodus provides Predictive Analytics services, including advisory, BI reporting components, tailor-made your analytics platform and managed services to help businesses benefit from a the most tangible insights. See how Synodus has been a trusted technology partner of clients in various fields solving their ambition & frustration with data. |
Wrapping Up
Predictive analytics will be a vital aspect for businesses in this data-driven age. There are many predictive analytics tools and vendors nowadays, including an affordable platform such as Excel. Company leaders should consider choosing a tool based on their needs, goals, resources, and budgets. Yet, predictive analytics in excel should be a short-term solution. In the long run, it does not bring tangible results.
More related posts from Big data blog you shouldn’t skip: